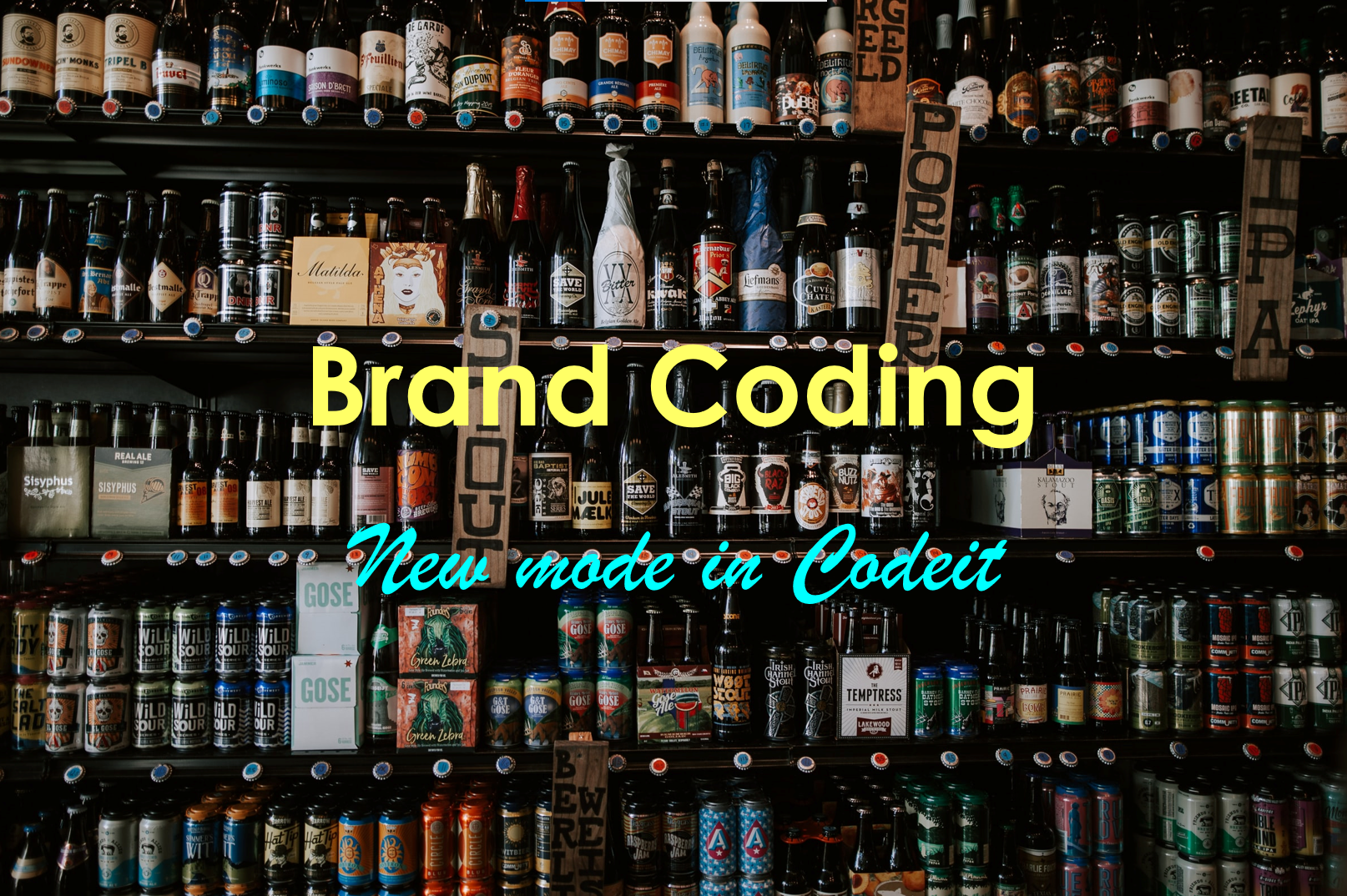
Guest Blog: Tim Brandwood, Digital Taxonomy
Thinking about all the brands of beer you know, what’s the first one that comes to mind? Heineken? Budweiser? Stella Artois? Doom Bar?!
I’ll bet you didn’t think of “Double Diamond”, “Skol” or “Party Seven”. Over time, the popularity of any given brand can rise and fall, so it is useful to track brand awareness and brand saliency. In a survey, when you ask an unprompted brand awareness question like the one above, you will generate a list of free text verbatim responses which then need to be analysed. If you’re just interested in getting a general feel for the data, you could view the data in a wordcloud. However, more often than not you’ll need to code the data to produce a more precise analysis.
Characteristics of Short Text
This type of data has three important defining characteristics:
- Repetitive – Short text responses from surveys tend to be quite repetitive. Lots of people will say the exactly the same thing, “Heineken”, for example.
- Typos – It’s very common for respondents to mistype answers so typos will be a big feature of the data, “Hienekin”, for example.
- Brand Variants – Responses can also mention brand variants and sub-brands of a main brand, “Heineken 0.0 Alcohol Free”, for example.
If you know in advance that your data will be like this, it influences the best approach to coding your data as quickly and efficiently as possible.
Hello Short Text Coding
To help tackle this kind of data, Codeit now has a brand new “Short Text” coding mode. This complements our existing coding modes Block Coding, Sequential Coding and Theme Explorer and adds a tool dedicated to the job of coding short, repetitive text items.
Distinct Items
Since short text is usually repetitive, it makes sense to display a list of distinct mentions rather than a full list of all the individual responses (like we do in Codeit’s “Block Coding Mode”). So, if “Heineken” is mentioned 15 times, then Codeit will just display this once with a count of 15. This is much more efficient as you can code multiple respondents (sometimes hundreds) by coding a single item of text in Codeit.

Fuzzy-Matching
To handle typos, the short text coding mode has an ability to fuzzy match text items and automatically group them together. For example, if we expand the “Heineken” group above, we can see that Codeit has automatically pulled in some misspelled items “Henieken” and “Hieneken”. This further increases the efficiency and speed of coding by increasing the number of items you can code in one “hit”.
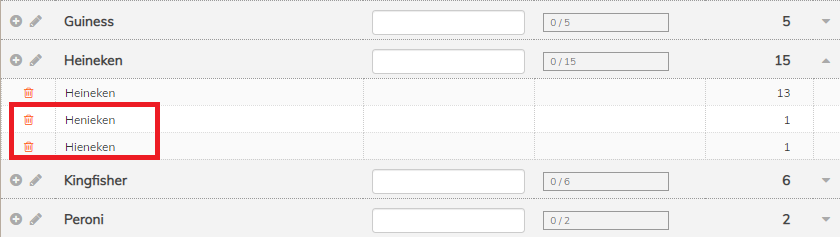
Merging
The fuzzy matching above is powerful and very useful, but it won’t catch everything. People still have a role to play in this process by applying human-level understanding and fine-tuning the automated suggestions Codeit generates. For example, suppose we have a number of mentions of “Carling” and “Carling black label”. Codeit may see these as separate items by default, but it’s very easy for a coder to multi-select the rows in Codeit and merge them into one group.
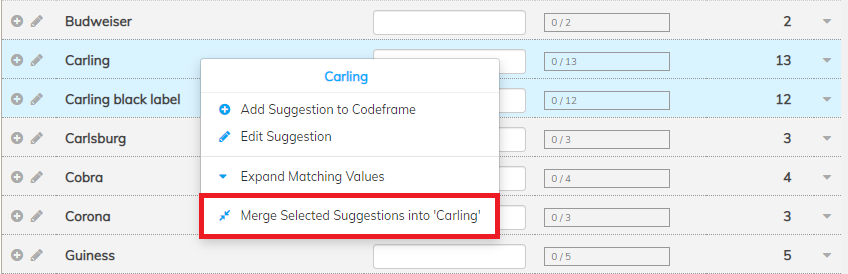
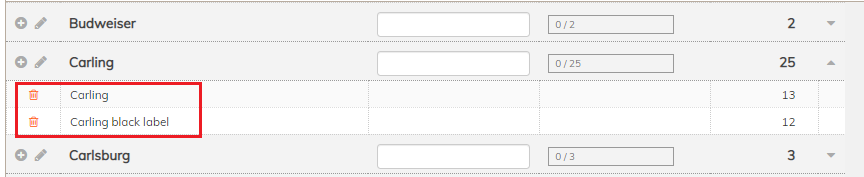
Miscellaneous
In this kind of data, there will always be a long tail of unique responses that don’t neatly fit into the automated suggestions. Again, this is where people are invaluable to the coding process. Codeit will group these together into a single “Unallocated” group, so a coder can quickly work through these and decide what action to take.
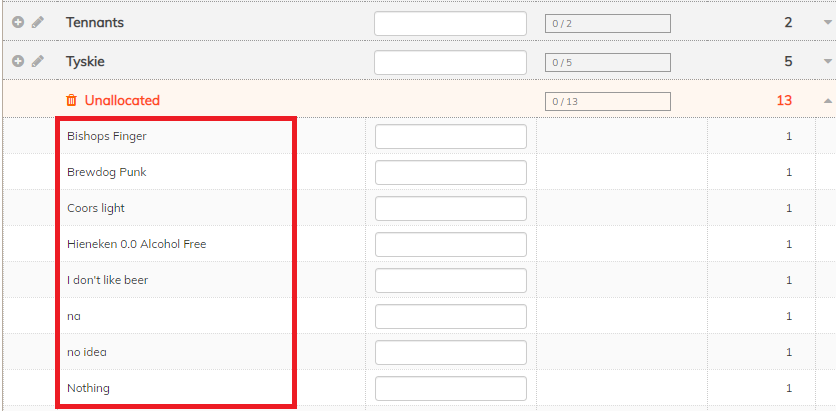
Full Control
Although Codeit is doing a lot of heavy lifting and speeding up the process considerably, the user is in full control. So at any time they can override any of the automated suggestions that Codeit makes. The short text coding tool has many features for moving items around, applying codes, renaming codes, editing the codeframe and so on. Short text coding is, like all the other tools in Codeit, all about the optimal combination of automated tools and human intervention, in order to achieve the best results.
Machine Learning
Lastly, as with all of our other coding modes, Codeit is continually learning by example. For example, once you show Codeit that “Carling black label” should be coded as “Carling” it will remember that and reapply that learning to future data. This means, when you re-use the learning on a project, or share the learning across projects, Codeit can autocode a large proportion of new data that comes into the system.
For on-going brand tracking projects, it’s very common to achieve rates of 85%-95% autocoding using Codeit.
Conclusion
Short text coding is quite a specific Use Case in the market research world, so we built a unique tool to meet this need. The short text coding tool in Codeit massively speeds up the process of coding short text, brands and so on, by blending the cutting edge automation with human level expert input.
For more details on short text coding or on Codeit in general then please contact your Askia key account manager.
Photo by Christin Hume on Unsplash